The Future of Intelligent Collaboration: Exploring Multi-Agent Systems and Exploratory AI
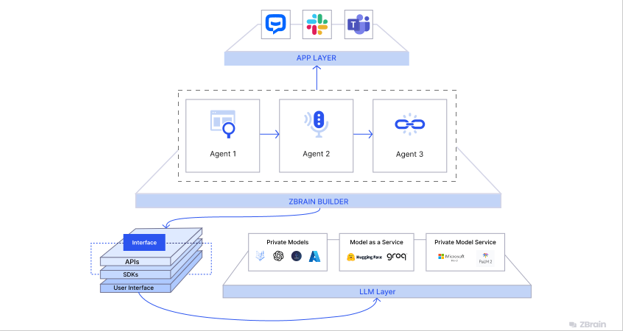
In an increasingly complex world where businesses need smarter, faster, and more adaptable systems to remain competitive, artificial intelligence (AI) is evolving beyond individual models. The era of multi-agent systems and exploratory AI is transforming how decisions are made and tasks are executed. Companies like ZBrain are leading this innovation by developing sophisticated platforms that enable machines to work in concert, think critically, and adapt dynamically.
One of the key advancements from ZBrain is the ZBrain Multi-Agent System, which showcases how interconnected agents can outperform single AI models by dividing tasks and collaboratively reaching goals. Another groundbreaking product, the ZBrain XPLR, redefines how we interact with data and AI, pushing the boundaries of real-time decision-making.
Let’s explore how these technologies work, their implications, and why they represent a turning point for AI-driven systems.
What Is a Multi-Agent System?
A multi-agent system (MAS) is a network of intelligent agents that interact with one another to achieve a common goal. Each agent in the system is autonomous, has a limited view of the environment, and specializes in certain tasks. However, when working together, these agents create an ecosystem capable of solving complex problems more efficiently than a single AI could manage alone.
MAS architecture is inspired by natural systems such as ant colonies and the human brain, where individual components work semi-independently but contribute to a greater, coordinated outcome. This distributed intelligence leads to better scalability, resilience, and adaptability—crucial features in dynamic business environments.
Inside the ZBrain Multi-Agent System
The ZBrain Multi-Agent System is a prime example of this next-generation AI infrastructure. ZBrain leverages a structured orchestration layer where multiple specialized agents handle tasks such as data analysis, workflow automation, content generation, and user interaction.
Each agent is designed with a specific function, yet is fully capable of communicating and negotiating with others. For example, one agent may collect and clean data, while another interprets patterns, and a third provides actionable insights or even creates summaries and visualizations.
Key advantages of ZBrain’s approach include:
- Task decomposition: Large, complex problems are broken down into manageable parts.
- Real-time collaboration: Agents share information instantly and adapt their strategy.
- Context awareness: Each agent can respond to changes without disrupting the whole system.
- Continuous learning: With feedback loops, agents refine their behavior over time.
This system allows enterprises to achieve results that are not only faster but also more accurate and contextually relevant.
What Makes Exploratory AI Different?
While most AI systems are built to respond to predefined queries or patterns, exploratory AI—as exemplified by ZBrain XPLR—takes a different approach. Instead of merely answering questions, it helps users ask better questions by exploring relationships in data, surfacing hidden patterns, and suggesting new angles.
ZBrain XPLR is a visual, interactive platform that enables teams to explore vast datasets and derive insights without needing advanced programming skills. This democratizes AI by putting powerful analytical tools into the hands of non-technical users such as marketers, executives, and analysts.
Notable features of exploratory AI through ZBrain XPLR include:
- Interactive data visualization: Dynamic graphs and charts update based on user input.
- Contextual intelligence: Recommendations adapt to the user’s actions and intent.
- Natural language interface: Users can interact with the system conversationally.
- Scenario testing: Explore “what-if” possibilities to guide strategic decisions.
This creates a more intuitive and engaging experience, helping users uncover insights they didn’t even know to look for.
Benefits of Combining Multi-Agent Systems with Exploratory AI
While each technology is powerful on its own, combining multi-agent systems with exploratory AI unlocks unprecedented potential.
- Faster Decision Making: Multi-agent systems automate the groundwork—data gathering, analysis, validation—while exploratory AI enables immediate, user-friendly interaction with the results.
- Scalability with Insight: The architecture can scale across departments or projects, while still tailoring outputs to specific user needs or business goals.
- Increased Innovation: By revealing novel insights and possibilities, these systems encourage more strategic thinking and innovation across teams.
- Reduced Bottlenecks: Technical dependencies are minimized, allowing business teams to act on insights without waiting on data teams or developers.
- Better Risk Management: Systems can proactively identify anomalies or potential risks and offer simulations or recommendations for mitigation.
Together, they represent a closed-loop intelligence system that can operate both autonomously and in concert with human users.
Real-World Applications and Industry Impact
The potential use cases for these technologies span across industries:
- Healthcare: From optimizing clinical workflows to discovering drug interactions.
- Finance: Detecting fraud in real-time, forecasting market trends, or managing portfolios.
- Retail: Personalizing customer experiences through behavior tracking and predictive modeling.
- Manufacturing: Managing supply chains, predictive maintenance, and quality control.
- Logistics: Route optimization, fleet management, and automated scheduling.
As businesses face increasing pressure to respond to real-time events, make informed decisions, and optimize operations, the adoption of systems like ZBrain’s becomes not just beneficial—but essential.
Challenges and Considerations
Despite their promise, implementing multi-agent and exploratory AI systems is not without challenges:
- Integration complexity: Merging these systems with legacy infrastructure can be difficult.
- Data quality: Poor data input leads to flawed output—garbage in, garbage out.
- Governance: It’s critical to ensure transparency, ethical alignment, and compliance.
- Change management: Organizations must adapt culturally and operationally to leverage these tools effectively.
Companies that prioritize user education, ethical standards, and system interoperability will gain the most from these technologies.
The Path Forward
ZBrain’s advancements illustrate a major evolution in AI: from isolated algorithms to cooperative ecosystems, and from reactive analytics to proactive exploration. By investing in multi-agent systems and exploratory AI, businesses can build smarter, more agile, and more resilient operations.
As AI continues to evolve, the organizations that embrace this shift early will be best positioned to lead—not just react—in the digital future.